Neural-Fly: the deep-learning algorithm that teaches drones to handle wind in all conditions
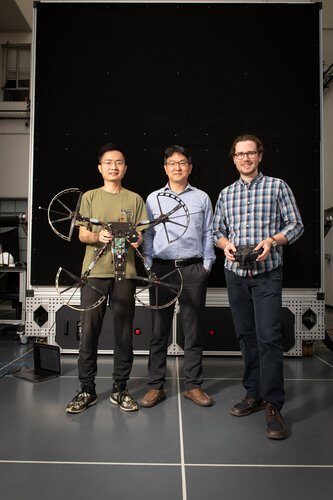
The algorithm in question has the ability to literally “train” the aircraft in just twelve minutes of flight time, in which an onboard computer records data in six different wind conditions. Neural-Fly is described in detail in the study published May 4 in Science Robotics and edited by author Soon-Jo Chung, Professor of Aerospace and Control and Dynamical Systems and Jet Propulsion Laboratory Research Scientist. The project originated and was tested inside the Caltech Center using a Real Weather Wind Tunnel, supported by more than 1,200 computer-controlled fans in order to simulate every minute detail. According to Chung, the unpredictability of wind and weather conditions has prompted scientists to change their approach and then going to prefer a method of deep learning, through which the drone would learn from previous experiences so as to be able to continuously adapt to flight conditions. Below is a video contribution: https://www.youtube.com/watch?v=TuF9teCZX0U
Neural-Fly, developed in collaboration with Anima Anandkumar, Bren Professor of Computer Science and Mathematical Sciences, and Yisong Yue, Caltech Professor of Computer Science and Mathematical Sciences, is based on systems previously known as Neural-Lander and Neural-Swarm. The former used a deep-learning method to track the drone‘s position and speed as it landed, so it could modify its landing trajectory and rotor speed to achieve the smoothest landing possible; Neural-Swarm, on the other hand, taught drones to fly autonomously in close proximity to each other.
A remarkable step forward, then, towards making uninhabited aerial vehicles (UAVs) safer and able to adapt to as many weather variables as possible.
Author: Guglielmo Maria Ruocco